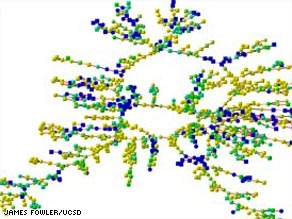
Image of the happiness network from CNN story.
The nodes are colored for average mood. Yellow is
happy, blue is sad, and green is in-between.
A networks blog at College of the Atlantic
After the discussion of the ecology papers in class on Friday I got curious about whether anyone had attempted to address the tendency of many people studying food web networks to treat them as undirected despite their directed nature. I spent some time doing some additional reading, and I found that the trend was pretty consistent through the papers that I found. The two papers that offered a justification for it (Williams et al., 2002; Dunne et al., 2002) both stated that a directed graph could be treated as undirected, because "effects can propagate through the network in either direction.". It seems to me that whether this is a valid assumption depends heavily on what exactly the network is being used to study. If one was studying the effects on the food web from the removal of certain species then perhaps it would be an okay idea to work under, because the effects of prey and predator removal would have similar effects on organisms throughout the food web. On the other hand if one was looking at transmission of toxins through an ecosystem then one would have to use a directed network to model it, because they are only going to move in one direction. In almost every other aspect of network analysis I would think that the directed nature of the food web is too much a key part of how the web works that it should not be ignored. There are perhaps a few situations in which an undirected food web could be applicable, but it seems to me that none of the articles that I found provided sufficient justification for an undirected food web.
A colleague of mine asked me why I didn't write this up as an official "Comment" for Science. My response was basically that I didn't think it would make a bit of difference if I did, and it's probably more useful to do it informally here, anyway. My intention is not to trash the Yu et al. paper, which as I mentioned above has a lot of genuinely good bits in it, but rather to point out that the common practices (i.e., regressions) for analyzing power-law distributions in empirical data are terrible, and that better, more reliable methods both exist and are easy to use. Plus, I haven't blogged in a while, and grumping about power laws is as much a favorite past time of mine as publishing bad power laws apparently is for some people.
a way of seeing the world that assumes that we are to information overload as fishes are to water: it's just what we swim in. Yiztak Rabin... has said that "if you have the same problem for a long time, maybe it's not a problem. Maybe it's a fact." That's information overload. Talking about information overload as if it explains or excuses anything is actually a distraction.... When you feel yourself getting too much information, ... don't say to yourself what happened to the information, but say to yourself what filter just broke? What was I relying on before that stopped functioning?
a natural question was how such research should be encouraged. Nobody on the panel seemed to make what I thought was an obvious point: one way to encourage such research is to make sure people across the sciences are well trained in mathematics and (theoretical) computer science. Interdisciplinary research depends on finding a common language, which historically has been mathematics, but these days more and more involves algorithms, complexity, and programming.
Theoretical computer science, and the algorithmic way of thinking, transcends our traditional boundaries. I believe that algorithms are relevant to every discipline of study, and will give eclectic examples from the arts and sciences to business and society. The examples span the spectrum from serious topics like protein folding and decoding Inka khipu to fun topics like juggling and magic.
There is only one machine.
The web is its OS.
All screens will look into the One.
No bits will live outside the One.
To share is to gain.
Let the One read it.
The One is us.